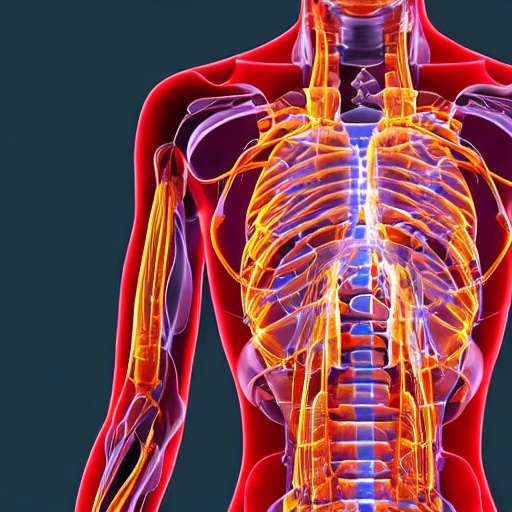
AI in Healthcare: Advancements and the Growing Threat to Cybersecurity
Artificial Intelligence (AI) has emerged as a revolutionary technology in the healthcare sector, offering unprecedented opportunities for enhanced diagnostics, personalized treatments, and improved patient outcomes. With the ability to analyze vast amounts of data and identify patterns that human physicians might overlook, AI has the potential to transform the way healthcare is delivered. However, as AI continues to evolve and become more integrated into healthcare systems, the need for robust cybersecurity measures becomes increasingly critical. This article explores the role of AI in healthcare and the accompanying cybersecurity challenges that demand attention.
The Rise of AI in Healthcare
AI has made significant strides in the healthcare industry, empowering healthcare professionals to make more accurate and timely diagnoses, develop tailored treatment plans, and predict patient outcomes. Machine learning algorithms can analyze large datasets, such as medical records, genomic information, and imaging scans, to identify patterns and anomalies that aid in disease detection and prognosis.
AI-powered diagnostic tools can sift through vast amounts of patient data, compare it to existing medical knowledge, and generate insights to support clinical decision-making. Additionally, AI algorithms can facilitate the interpretation of medical images, enabling more precise detection of abnormalities in radiology, pathology, and other imaging modalities. This technology holds the promise of reducing diagnostic errors and improving patient care.
Most AI experts believe that this blend of human experience and digital augmentation will be the natural settling point for AI in healthcare. Each type of intelligence will bring something to the table, and both will work together to improve the delivery of care.
For example, artificial intelligence is ideally equipped to handle challenges that humans are naturally not able to tackle, such as synthesizing gigabytes of raw data from multiple sources into a single, color-coded risk score for a hospitalized patient with sepsis.
Cybersecurity Challenges in AI-Enabled Healthcare
While the integration of AI in healthcare brings numerous benefits, it also introduces cybersecurity risks that can compromise patient privacy, data integrity, and the overall integrity of healthcare systems. Here are some key challenges:
Data Privacy and Patient Confidentiality
AI algorithms rely on vast amounts of sensitive patient data, including medical records, genetic information, and personal identifiers. Protecting patient privacy becomes paramount, as a breach in data security could lead to identity theft, financial fraud, or reputational damage.
The use of AI in healthcare also entails a risk of data security breaches, in which personal information may be made widely available, infringing on citizens’ rights to privacy and putting them at risk for identity theft and other types of cyberattacks. In July 2020, the New York based AI company Cense AI suffered a data breach that exposed highly sensitive data of upwards of 2.5 million patients who had suffered from car accidents, including such detailed information as names, addresses, diagnostic notes, dates and types of accident, insurance policy numbers and more. Although eventually secured, this data was briefly accessible to anyone in the world with an internet connection, underlining the very real danger of personal privacy breaches that patients are exposed to.
Lack of Transparency
A significant risk for AI is a lack of transparency concerning the design, development, evaluation,and deployment of AI tools. AI transparency is closely linked to the concepts of traceability and explainability, which correspond to two distinct levels at which transparency is required: 1) transparency of the AI development and usage processes (traceability), and 2) transparency of the AI decisions themselves (explainability).
Specific risks associated with a lack of transparency in biomedical AI include a lack of understanding and trust in predictions and decisions generated by the AI system, difficulties in independently reproducing and evaluating AI algorithms, difficulties in identifying the sources of AI errors and defining who and/or what is responsible for them, and a limited uptake of AI tools in clinical practice and in real-world settings.
Insider Threats
Healthcare organizations must be vigilant in managing access to AI systems, as insider threats can pose significant risks. Unauthorized access or misuse of AI systems by employees or contractors could lead to data breaches or the alteration of patient records.
Ethical and Legal Concerns
AI in healthcare raises ethical questions, such as the responsible use of patient data and the potential for algorithmic bias. Addressing these concerns requires a comprehensive legal framework to regulate the collection, storage, and use of patient data while ensuring transparency and accountability.
While artificial intelligence is presumed to be completely free of the social and experience-based biases entrenched in the human brain, these algorithms are perhaps even more susceptible than people to making assumptions if the data they are trained on is skewed toward one perspective or another.
There are currently few reliable mechanisms to flag such biases. “Black box” artificial intelligence tools that give little rationale for their decisions only complicate the problem – and make it more difficult to assign responsibility to an individual when something goes awry.
When providers are legally responsible for any negative consequences that could have been identified from data they have in their possession, they need to be absolutely certain that the algorithms they use are presenting all of the relevant information in a way that enables optimal decision-making.
An artificial intelligence algorithm used for diagnostics and predictive analytics should have the capability to be more equitable and objective than its human counterparts. Unfortunately, cognitive bias and a lack of comprehensive data can worm their way into AI and machine learning algorithms.
Mitigating Cybersecurity Threats
To effectively mitigate cybersecurity threats in AI-enabled healthcare, a multi-faceted approach is necessary:
Robust Security Infrastructure: Implementing robust security measures, including encryption, access controls, and secure authentication protocols, is crucial to safeguard patient data. Regular security assessments and audits can help identify vulnerabilities and address them promptly.
Staff Training and Awareness: Healthcare professionals and staff should receive comprehensive training on cybersecurity best practices to prevent accidental data breaches and understand the importance of data protection and privacy.
Regulatory Compliance: Healthcare organizations must comply with relevant data protection regulations, such as the General Data Protection Regulation (GDPR) and the Health Insurance Portability and Accountability Act (HIPAA), to ensure patient privacy and avoid legal repercussions.
Collaboration and Information Sharing: Encouraging collaboration between healthcare organizations, AI developers, and cybersecurity experts fosters knowledge exchange and promotes the adoption of best practices for secure AI integration.
Ethical AI Development: Developers should adhere to ethical guidelines when designing AI algorithms, ensuring transparency, fairness, and accountability in their decision-making processes. Regular audits and evaluations can help identify and rectify any biases or vulnerabilities in the AI system.
Conclusion
AI has immense potential to revolutionize healthcare, enabling accurate diagnoses, personalized treatments, and improved patient outcomes. However, the integration of AI in healthcare also poses significant cybersecurity challenges that must be addressed. By implementing robust security measures, fostering awareness, and adhering to ethical guidelines, healthcare organizations can leverage the benefits of AI while protecting patient privacy and maintaining the integrity of their systems. Entities using or selling AI-based health care products need to take into account federal and state laws and regulations applicable to the data they are collecting and using that govern the protection and use of patient information and other common practical issues that face AI-based health care products. A collaborative effort involving stakeholders from the healthcare, AI, and cybersecurity domains is vital to ensure a secure and transformative future for AI in healthcare.
Monitoring Remote Sessions
With more employees working from home, companies are seeking ways of monitoring remote sessions. One compelling case can be made for recording remote sessions for later playback and review. Employers are concerned that in the event of a security breach, they won’t be able to see what was happening on users’ desktops when the breach occurred. Another reason for recording remote sessions is to maintain compliance, as required for medical and financial institutions or auditing for business protocols, etc.
TSFactory’s RecordTS v6 will record Windows remote sessions reliably and securely for RDS, Citrix and VMware systems. Scalable from small offices with one server to enterprise networks with tens of thousands of desktops and servers, RecordTS integrates seamlessly with the native environment.
Click here to learn more about secure remote session recording.